Why you need an automated AML transaction monitoring solution
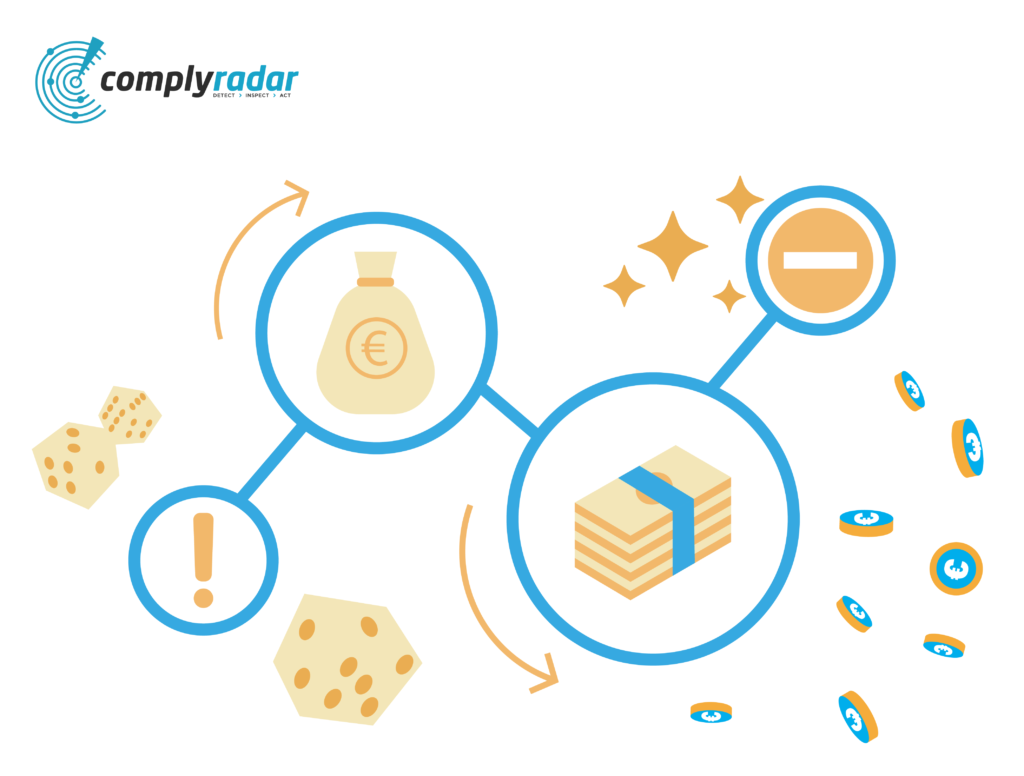
We’re living in the digital age, meaning we’re all too familiar with how the online sphere has dominated the world of financial transactions, particularly in recent years. Naturally, a consequence of this is that cybercrime and online money laundering have risen exponentially. Automating your AML transaction monitoring process, rather than doing it manually, will give […]
What is AML transaction monitoring?
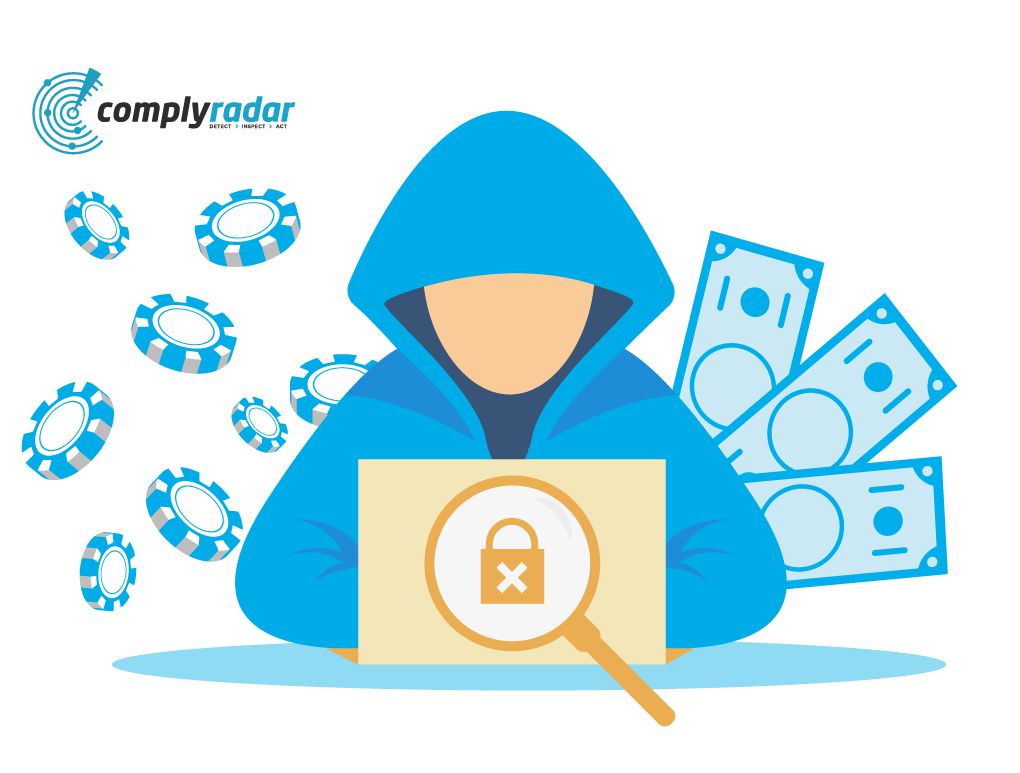
Even if you’re not directly involved in the Banking & Finance or Gambling & iGaming sectors, there’s a pretty good chance that you’ve at least heard – or read – about Anti-Money Laundering (AML). It refers to a set of laws, regulations, and procedures intended to prevent criminals from disguising illegally obtained funds as legitimate […]
Using AI and machine learning to fight money laundering
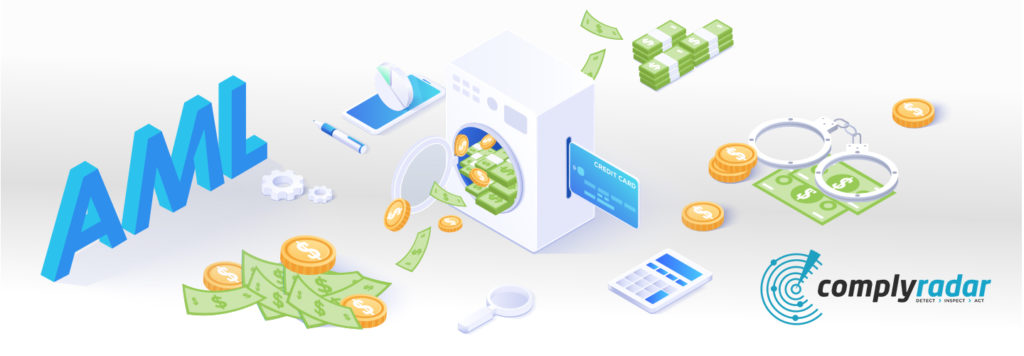
Data scientists have done a great deal for us in the way of making our financial lives easier and, without a doubt, more secure than ever before. These advancements aren’t always used for good, however. While technology has brought the financial world forward in leaps and bounds, it’s worth remembering that this same technology is […]